Introduction
With the rise of AI chatbots on the market, it is essential to identify their biases early during the design and development phases. AI systems are not immune to reflecting and perpetuating societal biases, and without proper accountability, they can inadvertently discriminate against certain individuals or communities. As AI chatbots become more sophisticated, it is essential to maintain transparency around how the model was constructed and the data it is trained on. Doing this helps reduce the risk of bias or discrimination while also guaranteeing that everything functions as intended and any issues are addressed promptly. To do this, make sure the data used for training models are not only diverse but also thoroughly vetted to prevent any unwanted prejudices.
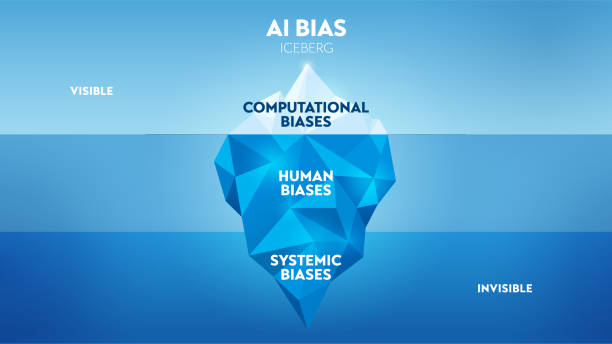
Understanding Bias and Discrimination in AI Chat Assistants
Bias and discrimination in AI chat assistants refer to the potential for these systems to exhibit unfair or discriminatory behavior towards certain individuals or groups based on factors such as race, gender, age, or cultural background. While AI chat assistants are designed to assist users and provide helpful information, they can inadvertently reflect and perpetuate societal biases that exist in the training data or the algorithms that underpin their functioning. Understanding bias and discrimination in AI chat assistants is crucial for identifying, addressing, and mitigating these issues. Here are key points to consider:
Implicit Bias
AI chat assistants learn from large datasets, which can inadvertently contain biases present in society. These biases may be reflected in the responses, recommendations, or decisions made by the chat assistant. Implicit biases, which are unconscious or unintentional biases, can be unintentionally learned and perpetuated by AI systems.
Stereotyping and Prejudice
AI chat assistants can inadvertently reinforce stereotypes or engage in prejudiced behavior. This can occur when the system’s responses or actions are based on generalizations or assumptions about certain groups or individuals, leading to discriminatory outcomes or unequal treatment.
Representation Bias
Bias can arise from underrepresentation or misrepresentation of certain groups in the training data. If the data used to train the AI chat assistant lacks diversity or includes skewed proportions of different demographic groups, it can result in biased outcomes that disproportionately affect marginalized communities.
Feedback Loop Bias
AI chat assistants interact with users and learn from their feedback. If user interactions contain biased or discriminatory inputs, the chat assistant may inadvertently reinforce and amplify those biases in its subsequent responses or recommendations. This feedback loop can perpetuate biased behavior over time.
Contextual Bias
AI chat assistants may struggle to understand or appropriately respond to nuanced cultural or contextual factors. The inability to recognize or adapt to different cultural norms, languages, or dialects can result in biased or inappropriate responses, leading to feelings of exclusion or discrimination for certain users.
Lack of Diversity in Development Teams
The lack of diversity within the development teams responsible for creating AI chat assistants can contribute to bias.
Homogeneous teams
Homogeneous teams may inadvertently overlook or fail to recognize biases that affect individuals from different backgrounds, resulting in biased outcomes in the system’s behavior.
Unintentional Discrimination
Discrimination in AI chat assistants is not always intentional. Biased outcomes can occur due to the systemic nature of biases present in the training data, algorithmic design choices, or the lack of robust mechanisms to address biases during development and deployment.
Components of a Bias and Discrimination Accountability Framework
A comprehensive bias and discrimination accountability framework for AI chat assistants should include various components that work together to identify, mitigate, and address biases and discriminatory behavior. These components ensure transparency, fairness, and accountability in the design, development, and deployment of AI systems. Here are key components to consider when implementing such a framework:
Bias Identification and Evaluation
The framework should include mechanisms to identify and evaluate biases in AI chat assistants. This involves conducting regular audits, reviewing system behavior, and leveraging external evaluation processes. Bias assessment metrics and techniques, such as fairness indicators, can be employed to detect and measure biases accurately.
Transparent Decision-Making and Explainability
The accountability framework should promote transparency in the decision-making processes of AI chat assistants. Users should have access to explanations regarding the factors influencing the system’s responses or recommendations. Incorporating explainable AI techniques, such as interpretable algorithms and model-agnostic approaches, enhances transparency and user understanding.
Dataset Curation and Bias Mitigation
To address data bias, the framework should emphasize responsible dataset curation. Training datasets should be diverse, representative, and free from biased or discriminatory content. Bias mitigation techniques, including data augmentation, debiasing algorithms, and adversarial training, can be employed to reduce biases in AI chat assistants.
Continuous Monitoring and Auditing
Regular monitoring and auditing are critical to ensure ongoing accountability. Real-time monitoring of AI chat assistant interactions, user feedback analysis, and performance evaluations help detect biases and discriminatory behavior. Independent audits by external experts or third-party organizations can provide unbiased assessments of the system’s fairness and performance.
User Feedback and Redress Mechanisms
Creating channels for user feedback and redress is essential in holding AI chat assistants accountable. Users should be able to report biases, discriminatory behavior, or concerns. Feedback mechanisms, such as user surveys, public consultations, or dedicated support channels, should be established to gather insights and address user grievances effectively.
Governance and Responsible AI Practices
The accountability framework should outline governance practices and responsible AI principles that guide the development and deployment of AI chat assistants. This includes ethical guidelines, diversity considerations, and the establishment of multidisciplinary teams to oversee AI system development, deployment, and monitoring.
Bias-Aware Training and Evaluation
Integrating bias awareness into the AI chat assistant’s training process is crucial. This involves creating diverse training scenarios, including examples that challenge biases, and monitoring the system’s behavior during training. Evaluation metrics, such as demographic parity or equalized odds, can be used to assess the system’s performance with respect to bias and fairness.
External Auditing and Certification
Collaboration with external organizations for independent auditing and certification can enhance accountability. These organizations can evaluate AI chat assistants against predefined fairness and non-discrimination standards, providing an external validation of the system’s performance and fairness.
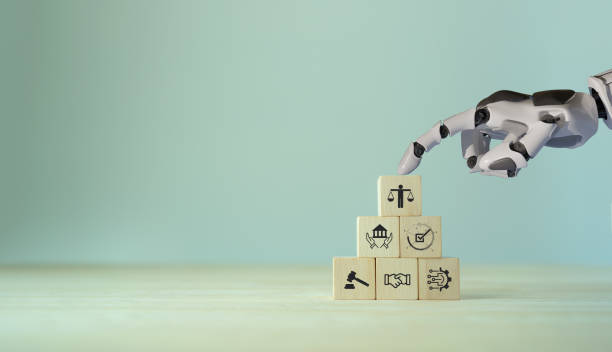
Challenges in Creating a Bias and Discrimination Accountability Framework
Creating a bias and discrimination accountability framework for AI chat assistants is a complex task that involves addressing various challenges. These challenges arise due to the intricacies of bias detection, the dynamic nature of AI systems, and the need to strike a balance between different stakeholder perspectives. Here are some key challenges to consider:
Subjectivity and Contextual Understanding
Identifying and addressing biases in AI chat assistants requires a deep understanding of the context and the subjective nature of biases. Bias detection involves interpreting nuances in language, cultural references, and social dynamics, which can be challenging due to the diverse range of users and their individual perspectives.
Lack of Clear Guidelines and Standards
The field of AI ethics and bias mitigation is still evolving, and there is a lack of clear and universally accepted guidelines or standards for addressing biases in AI systems. The absence of a unified framework makes it difficult to establish consistent practices and metrics for measuring bias.
Complex Data Ecosystems
AI chat assistants rely on vast and diverse datasets, which may introduce biases through the inclusion of historical or societal prejudices. Managing and curating these datasets to mitigate biases requires significant effort, especially considering the large-scale data ecosystems involved in AI systems.
Interplay between Algorithmic Complexity and Bias
AI chat assistants often utilize complex algorithms that can introduce unintended biases due to the intricate interplay of data, training methods, and model architectures. Understanding and addressing biases in these algorithms is challenging, as biases can emerge from complex interactions that are difficult to disentangle and quantify.
Intersectionality and Multiple Dimensions of Bias
Bias is not limited to a single dimension; it can manifest at the intersection of multiple social identities, such as race, gender, and age. Capturing and addressing intersectional biases in AI chat assistants requires nuanced approaches that account for the complex interdependencies between different forms of bias.
Limited Representation and Diversity in Development Teams
The lack of diversity and representation in AI development teams can inadvertently perpetuate biases. Homogeneous teams may overlook or fail to recognize biases that affect individuals from different backgrounds. Ensuring diverse perspectives within development teams is crucial for identifying and addressing biases effectively.
Balancing Fairness and Accuracy
Striving for fairness in AI chat assistants can sometimes conflict with accuracy. In an attempt to address biases, there is a risk of compromising the overall accuracy and effectiveness of the system. Achieving a balance between fairness and accuracy is a challenge that requires careful consideration and trade-offs.
Continuous Monitoring and Evaluation
Monitoring and evaluating the performance of AI chat assistants for biases is an ongoing task. Detecting emerging biases and adapting the system accordingly requires continuous monitoring, regular audits, and robust evaluation methodologies. Maintaining a dynamic framework that can evolve with changing user needs and societal dynamics is a challenge.
Lack of User Understanding and Engagement
Users may not always be aware of biases in AI chat assistants or have a clear understanding of how they can provide feedback or seek redress. Bridging the gap between users and developers, fostering user understanding and engagement, and creating accessible channels for feedback are challenges in ensuring user participation in the accountability framework.
Ethical Considerations and Unintended Consequences
Addressing biases involves navigating complex ethical considerations. While efforts are made to mitigate biases, unintended consequences can still arise, such as overcorrection or the introduction of new biases. Anticipating and mitigating these unintended consequences is a challenge in the design and implementation of the accountability framework.
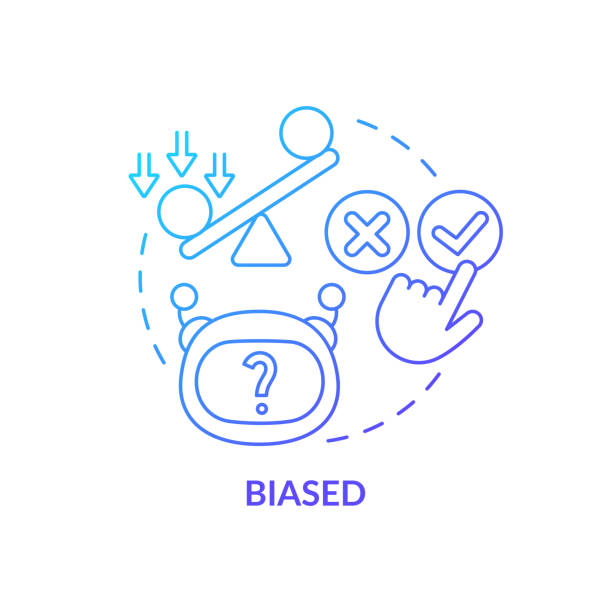
Best Practices for Implementing a Bias and Discrimination Accountability Framework
Implementing a bias and discrimination accountability framework for AI chat assistants requires a thoughtful and systematic approach. To ensure the effectiveness of the framework, it is essential to follow best practices that promote transparency, fairness, and accountability. Here are some best practices for implementing such a framework:
Define Clear Goals and Objectives
Establish clear goals and objectives for the bias and discrimination accountability framework. Define the specific biases and forms of discrimination you aim to address and articulate the desired outcomes. This clarity will guide the development and evaluation of the framework.
Foster Diversity and Inclusion
Promote diversity and inclusion within the development teams responsible for AI chat assistants. Ensure representation from diverse backgrounds and perspectives, including gender, race, ethnicity, and cultural diversity. This diversity helps identify and mitigate biases and enhances the overall fairness of the system.
Establish Ethical Guidelines
Develop and adhere to comprehensive ethical guidelines that address bias and discrimination in AI chat assistants. These guidelines should align with established ethical principles, human rights standards, and anti-discrimination laws. Consider incorporating guidelines such as the Fairness, Accountability, and Transparency (FAT) principles or similar frameworks.
Conduct Bias Assessments and Audits
Regularly assess and audit AI chat assistants to identify and evaluate potential biases. Conduct bias assessments using appropriate techniques such as fairness indicators, statistical analyses, or external audits by independent experts. These assessments help identify areas of improvement and ensure ongoing accountability.
Mitigate Biases in Training Data
Take proactive steps to mitigate biases in the training data. Curate diverse and representative datasets that accurately reflect the user population. Implement data augmentation techniques, data balancing approaches, or debiasing algorithms to reduce biases present in the training data and prevent the perpetuation of unfair patterns.
User Feedback and Redress Mechanisms
Establish clear channels for user feedback and provide mechanisms for users to report biases or discriminatory behavior. Create accessible and user-friendly feedback mechanisms such as user surveys, dedicated support channels, or public consultations. Respond to user concerns promptly and transparently, and take appropriate measures to address reported biases.
Regular Training and Education
Provide regular training and education to the development teams involved in creating AI chat assistants. Ensure that developers and data scientists are aware of the ethical considerations and potential biases in AI systems. Foster a culture of continuous learning and keep teams updated on the latest research and best practices in bias mitigation.
Collaborate with External Experts and Auditors
Engage external experts, auditors, or third-party organizations to conduct independent assessments of the AI chat assistants. Collaborate with individuals or groups specializing in bias mitigation, ethics, or social impact to provide unbiased evaluations and recommendations for improvement.
Iterate and Improve
Implement a process of continuous improvement and iteration for the bias and discrimination accountability framework. Regularly evaluate the framework’s effectiveness, learn from experiences, and incorporate feedback from users, external experts, and stakeholders. Stay informed about emerging research, industry best practices, and evolving regulatory requirements to keep the framework up to date.
Conclusion
Creating a bias and discrimination accountability framework for AI chat assistants is crucial to ensure fair, unbiased, and responsible interactions between AI systems and users. By addressing challenges related to data bias, explainability, diversity, and evolving societal norms, organizations can implement a robust framework that promotes transparency, fairness, and accountability. The key components of such a framework include bias identification and evaluation, transparent decision-making, dataset curation, continuous monitoring, user feedback mechanisms, responsible AI practices, and external auditing.